
In October, Box unveiled a new pricing approach for the company’s generative AI features.
Instead of a flat rate, the company designed a unique consumption-based model.
Each user gets 20 credits per month, good for any number of AI tasks that add up to 20 events, with each task charged a single credit.
If the customer surpasses that, it would be time to have a conversation with a salesperson about buying additional credits.
Spang says, for starters, that in spite of the hype, generative AI is clearly a big leap forward, and software companies need to look for ways to incorporate it into their products.
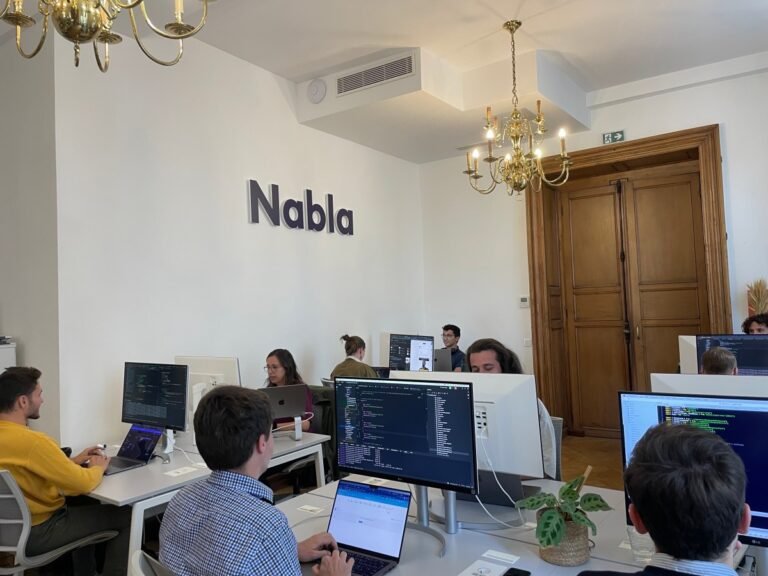
Nabla has been working on an AI copilot for doctors and other medical staff.
Nabla then uses a large language model refined with medical data and health-related conversations to identify the important data points in the consultation — medical vitals, drug names, pathologies, etc.
The company uses a combination of an off-the-shelf speech-to-text API from Microsoft Azure and its own speech-to-text model (a refined model based on the open-source Whisper model).
Once the LLM has processed the transcript, Nabla de-pseudonymizes the output.
However, doctors can give their approval and ask for the patient consent to share medical notes with Nabla so that they can be used to correct transcription errors.

Google’s DeepMind Robotics researchers are one of a number of teams exploring the space’s potential.
The newly announced AutoRT is designed to harness large foundational models, to a number of different ends.
In a standard example given by the DeepMind team, the system begins by leveraging a Visual Language Model (VLM) for better situational awareness.
A large language model, meanwhile, suggests tasks that can be accomplished by the hardware, including its end effector.
LLMs are understood by many to be the key to unlocking robotics that effectively understand more natural language commands, reducing the need for hard-coding skills.

Arkon Energy, a data center infrastructure company, closed a $110 million private funding round to expand its operations, the company’s CEO Josh Payne shared exclusively with TechCrunch.
“These sites appeal to both bitcoin miners and AI [or] machine learning clients who have very high power computing demands,” Payne said.
“We are essentially a landlord who owns the underlying infrastructure assets.”Arkon’s business model focuses on strategically acquiring distressed data center assets across the globe.
“The current and future demand for data center capacity of all types that we are seeing globally, but especially in the U.S., is unprecedented and monumental.
Arkon aims to fill that gap by providing the underlying infrastructure layer that the AI sector relies on.
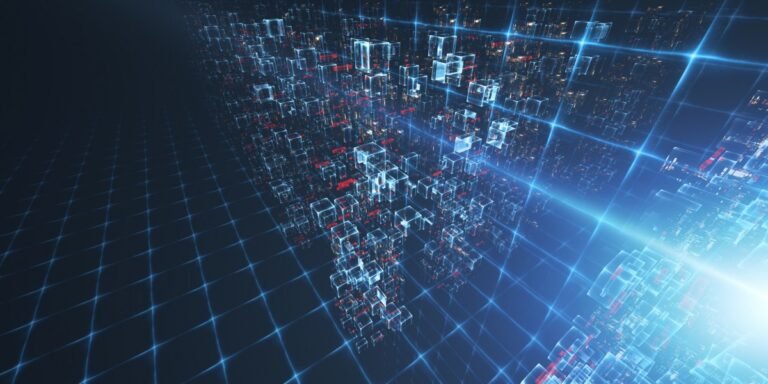
Akron Energy, a data center infrastructure company, closed a $110 million private funding round to expand its operations, the company’s CEO Josh Payne shared exclusively with TechCrunch.
“These sites appeal to both bitcoin miners and AI [or] machine learning clients who have very high power computing demands,” Payne said.
“We are essentially a landlord who owns the underlying infrastructure assets.”Akron’s business model focuses on strategically acquiring distressed data center assets across the globe.
“The current and future demand for data center capacity of all types that we are seeing globally, but especially in the U.S., is unprecedented and monumental.
Akron aims to fill that gap by providing the underlying infrastructure layer that the AI sector relies on.

The plan is for “centers of excellence” to be set up to support the development of dedicated AI algorithms that can run on the EU’s supercomputers, they added.
AI startups are more likely to be accustomed to using dedicated compute hardware provided by US hyperscalers to train their models than tapping the processing power offered by supercomputers as a training resource.
Using its supercomputing resources to fire up AI startups specifically has emerged as a more recent strategic priority after the EU president’s announcement of the compute access for AI model training program this fall.
It’s still early days for the EU’s ‘supercompute for AI’ program so it’s unclear whether there’s much model training upside to report off of dedicated access as yet.
But the early presence of Mistral in the EU’s supercomputing access program may suggest an alignment in the thinking.

OpenAI is expanding its internal safety processes to fend off the threat of harmful AI.
In-production models are governed by a “safety systems” team; this is for, say, systematic abuses of ChatGPT that can be mitigated with API restrictions or tuning.
Frontier models in development get the “preparedness” team, which tries to identify and quantify risks before the model is released.
So, only medium and high risks are to be tolerated one way or the other.
For that reason OpenAI is making a “cross-functional Safety Advisory Group” that will sit on top of the technical side, reviewing the boffins’ reports and making recommendations inclusive of a higher vantage.

OpenAI formed the Superalignment team in July to develop ways to steer, regulate and govern “superintelligent” AI systems — that is, theoretical systems with intelligence far exceeding that of humans.
Superalignment is a bit of touchy subject within the AI research community.
“I think we’re going to reach human-level systems pretty soon, but it won’t stop there — we’re going to go right through to superhuman systems … So how do we align superhuman AI systems and make them safe?
But the approach the team’s settled on for now involves using a weaker, less-sophisticated AI model (e.g.
Well, it’s an analogy: the weak model is meant to be a stand-in for human supervisors while the strong model represents superintelligent AI.

Instead, what is immediately changing as a result of this ruling is the legality surrounding the app store business model itself — and potentially others.
“What we know right now is that this is going to impact the walled garden business model Google and Apple and other companies have enjoyed for a while,” Swanson said.
In fact, the legal risk from this business model may encourage other businesses to change, even without being dragged to court.
Apple didn’t regularly engage in side deals (though it considered one with Netflix) nor did it pay developers to launch on its app store instead of theirs, as Apple only offers one route to app distribution: the App Store.
“Just because it is your business model does not mean it is legal or that it’s right,” VanMeter pointed out.

Google’s making the second generation of Imagen, its AI model that can create and edit images given a text prompt, more widely available — at least to Google Cloud customers using Vertex AI who’ve been approved for access.
Text and logo generation brings Imagen in line with other leading image-generating models, like OpenAI’s DALL-E 3 and Amazon’s recently launched Titan Image Generator.
These techniques also enhance Imagen 2’s multilingual understanding, Google says — allowing the model to translate a prompt in one language to an output (e.g.
Google didn’t reveal the data that it used to train Imagen 2, which — while disappointing — doesn’t exactly come as a surprise.
Instead, Google offers an indemnification policy that protects eligible Vertex AI customers from copyright claims related both to Google’s use of training data and Imagen 2 outputs.