The field of artificial intelligence (AI) is constantly evolving and changing, as new techniques are developed and embraced by those in the industry. One notable trend that has emerged in recent years is the incorporation of AI into numerous fields, including healthcare, transportation, and marketing.
This growing use of AI has led to a number of important challenges that must be addressed if these systems are to be successful. For example, creating accurate models that account for human behavior can be difficult – especially when data from different sources is being combined. Furthermore, widespread adoption of AI will require a robust safety net should things go wrong; currently there are few protections in place for individuals who may become negatively impacted by AI technology.
Overall, Stanford’s report provides an overview of both the dangers and possibilities posed by artificial intelligence. While there are many challenges ahead for this nascent industry, it holds great potential to transform many aspects of our lives
It is not clear what AI will ultimately be capable of, but one thing is for sure: the technology is moving forward at a rapid pace. This survey finds that almost half of industry experts believe that AI will takeover some or all human jobs within the next several years. While this may seem far-fetched, it is important to remember that AI has already made significant inroads into other areas such as computer decisionmaking and language recognition. If the trend continues, there is no telling what miraculous feats AI might accomplish in the future.
In light of the rapid development of artificial intelligence and its implications for society, it is important to consider ways in which AI can be effectively implemented. One approach would be to create public opinion support for AI through education programs, creating an understanding among the general public about the potential benefits and risks of this technology. While there are still many unresolved questions surrounding AI, new analysis included in this year’s report provides valuable insight into how it is being developed and how it might impact future generations.
So, in the end, all you really need to create a successful blog is a good idea and some help from others. When starting out, focus on creating content that is valuable and interesting to your readers, because they are likely to return over and over again if
- AI development has flipped over the last decade from academia-led to industry-led, by a large margin, and this shows no sign of changing.
- It’s becoming difficult to test models on traditional benchmarks and a new paradigm may be needed here.
- The energy footprint of AI training and use is becoming considerable, but we have yet to see how it may add efficiencies elsewhere.
- The number of “AI incidents and controversies” has increased by a factor of 26 since 2012, which actually seems a bit low.
- AI-related skills and job postings are increasing, but not as fast as you’d think.
- Policymakers, however, are falling over themselves trying to write a definitive AI bill, a fool’s errand if there ever as one.
- Investment has temporarily stalled, but that’s after an astronomic increase over the last decade.
- More than 70% of Chinese, Saudi, and Indian respondents felt AI had more benefits than drawbacks. Americans? 35%.
Given that these products are marketed to everyday people, it is important to examine how well they actually perform. The report provides a detailed look at each product and how it fares in various areas. While some may be more effective than others, all are worth considering if you’re looking for an easy way to improve your fitness routine or overall health.
Technical AI ethics are important because they help define the boundaries of what can and cannot be done with artificial intelligence technologies. By understanding these boundaries, it is possible to create safe and ethical applications of AI technology.
When designing machine learning models, it’s important to be aware of the bias and toxicity potential present in these models. Unfiltered models are much easier to lead into problematic territory – instruction tuning, which is adding a layer of extra prep (such as a hidden prompt) or passing the model’s output through a second mediator model, is effective at improving this issue, but far from perfect.
The increase in “AI incidents and controversies” alluded to in the bullets is best illustrated by this diagram: As artificial intelligence (AI) continues to evolve, so too does the potential for malfunctions or accidents. In December 2017, a self-driving Uber car ran over a woman crossing the road in Arizona, leading to public scrutiny of how autonomous technology should be handled. And earlier this year, reports surfaced of Google’s AI malfunction
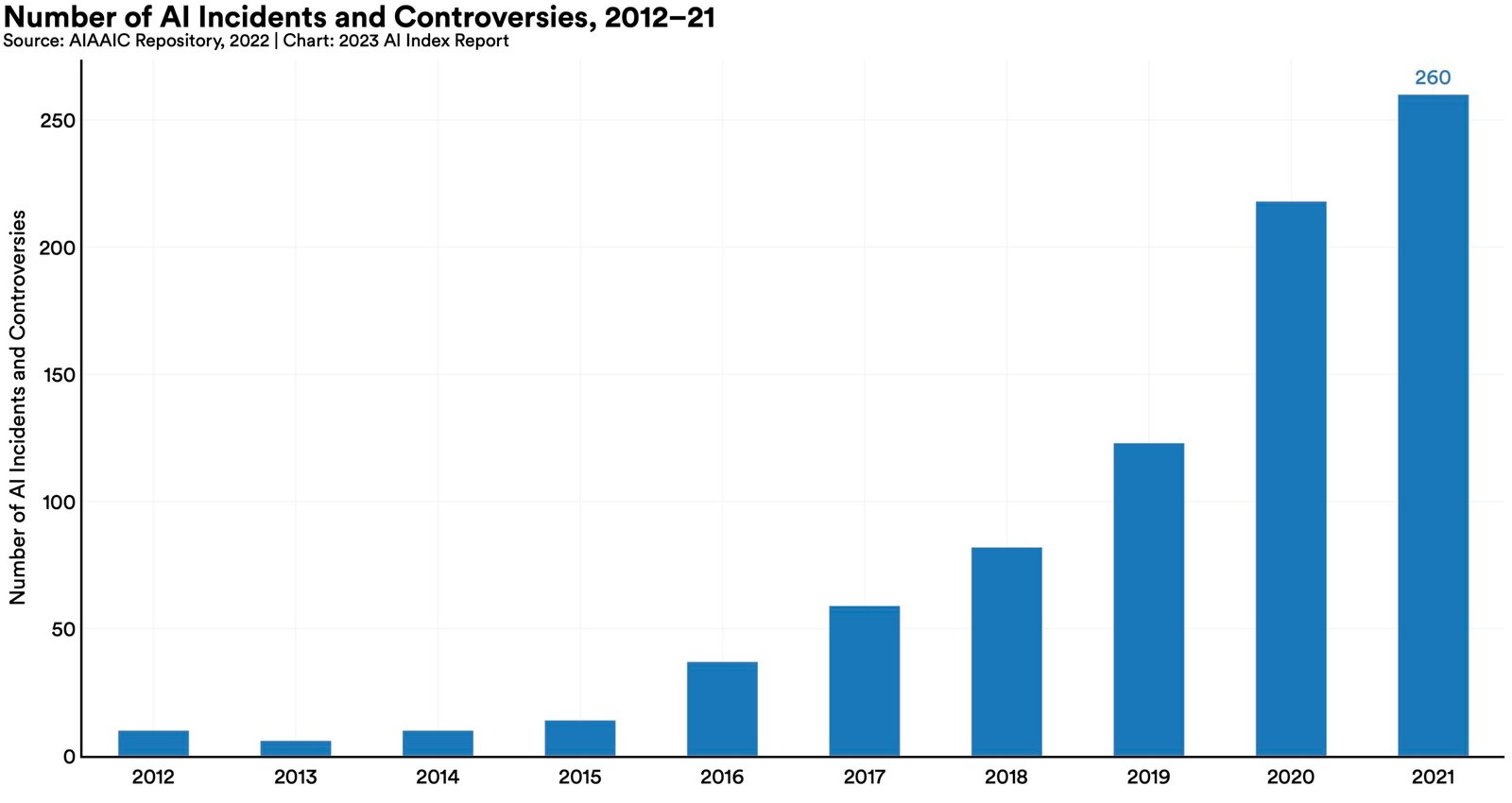
The Stanford HAI dataset is a largecollection of social media posts that have been tagged with terms such as ‘happy’, ‘sad’, and ‘
The trend of ChatGPT and other large language models is upwards. This was before the mainstream adoption of such models, as well as the vast improvement in image generators. You can be sure that there will be an even bigger increase in the future because more people are using these tools.
Including variables that are unrelated to the outcome of a model, such as race or sex, can lead to inaccurate predictions. For example, if a model is designed to predict how likely someone is to vote in an upcoming election, including information about their race or sex could inaccurately determine who actually votes. Additionally, models that are not fair may be less credible and may cause organizations not to adopt them.
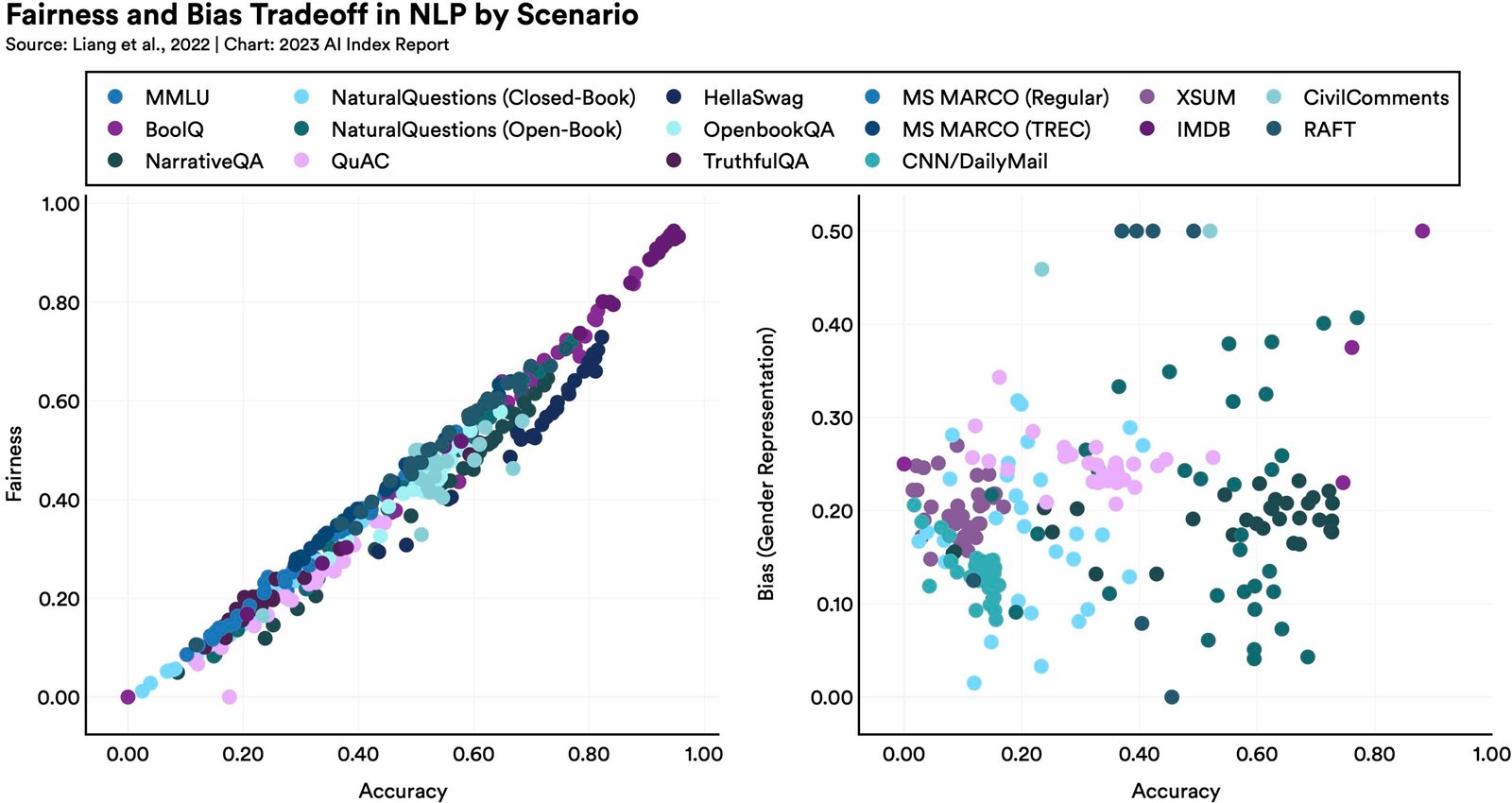
Robert Calvert is one of the most accomplished submariners alive. He has served in the Navy for more than three decades and as a result, has logged
As the report notes, language models which perform better on certain fairness benchmarks tend to have worse gender bias. This is likely because these models are optimizations of existing linguistic structures and frameworks, which may inadvertently favor specific genders or perspectives. However, it’s also possible that this disparity is due to inherent inefficiencies in these approaches when it comes to accurately representing or accounting for gender differences in language use. Until we can better understand how these large-scale machine learning approaches work, it’ll be hard to make any significant strides towards improving their accuracy andclusivity overall.
One of the key challenges for AI tasked with fact-checking is that the task is difficult and there are a lot of sources of information to evaluate. This makes it difficult for AI to distinguish reliable information from misinformation, which can lead to inaccurate evaluations. One way to combat this problem is by using machine learning algorithms, which can help identify reliable sources and identify any bias in the source. However, so far these techniques have been unsuccessful in improving accuracy overall. There are also a number of other factors that need to be considered when evaluating information, such as context and reliability. Ultimately, while fact-checking may seem like a straightforward task, it remains one that requires significant human oversight because computers cannot always tell the difference between true and false statements
Many people are concerned about the increasing use of artificial intelligence, especially as it pertains to how it can affect our personal data. There is a lot of talk about fairness, accountability, and transparency when it comes to AI, and there is definitely a concern that we not only need to be studying these issues more closely but are also quickly running into a number of challenges in regard to how they should be addressed. One such challenge is ensuring that people have confidence in the way AI behaves – if they don’t feel like their data is being used fairly or responsibly then it’s hard for them to trust its results. This has led to an increase in submissions at conferences on these topics as well as at NeurIPS issues like fairness, privacy, and interpretability. It’s important that we continue exploring theseissues so that we can make sure that AI benefits everyone involved – both now and in the future
Looking at the data outlined in this paper, it is clear that Health Analysis Institute (HAI) has done a great job of summarizing and organizing highlights from recent scientific studies. By providing concise summaries of each study, HAI has made it easy for readers to get a general idea of what was studied and its implications. Furthermore, by breaking down the research into different subsections, users are able to explore in more detail any particular topic they are interested in. Overall, this paper is an excellent resource for anyone looking to better understand recent scientific advancements pertaining to health statistics.